Objectives
To build computational framework that leverages NLP
- Structure unstructured notes
- Foundation to build Analytics Platform
Per Patient
- Extract the timeline of clinical events from notes using NLP
- Stage Info, Time of Tumor Progression and other clinical information
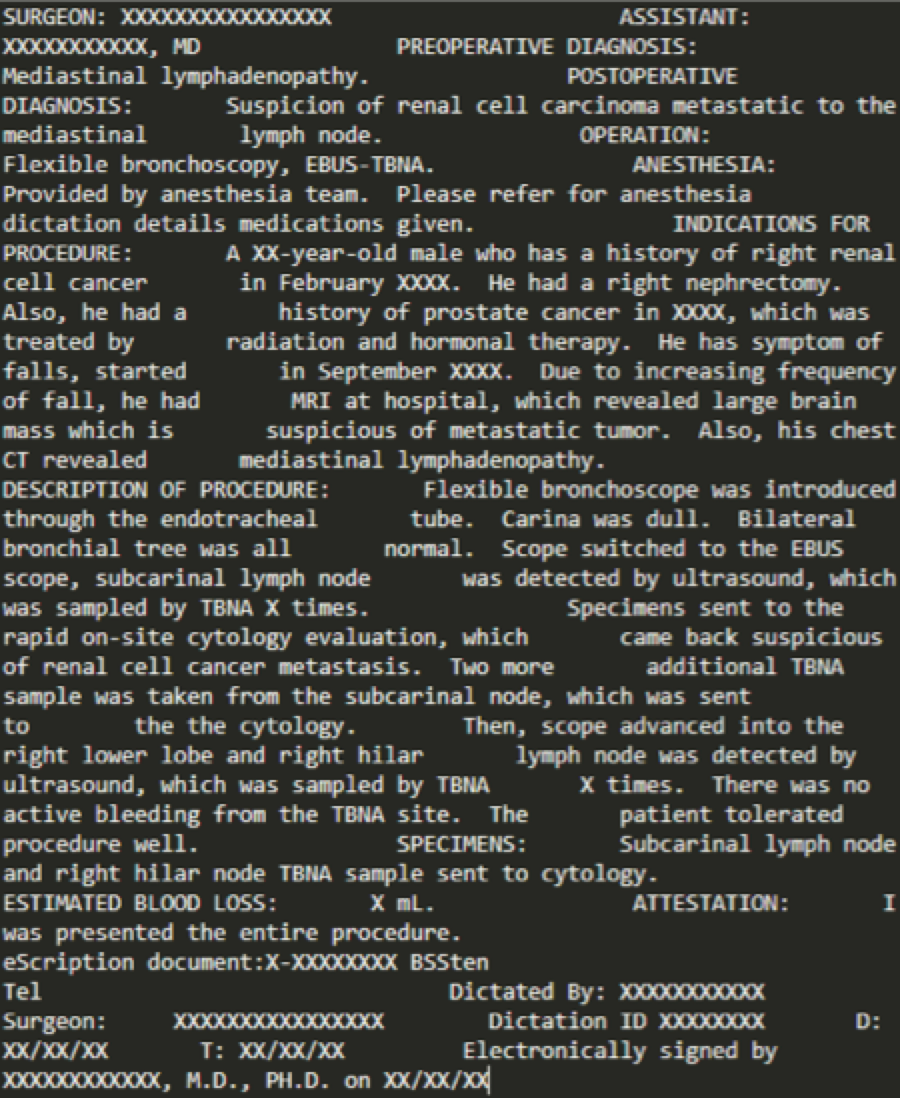
Workflow
Header Identification:
Section Identification:
Event Extraction:
Data Visualization:
Project Wrap-up:
Clustering & structure-based method
Rule-based method & classification
Obtain clinical events from sections
Flask web framework
Build an easy-to-use python library
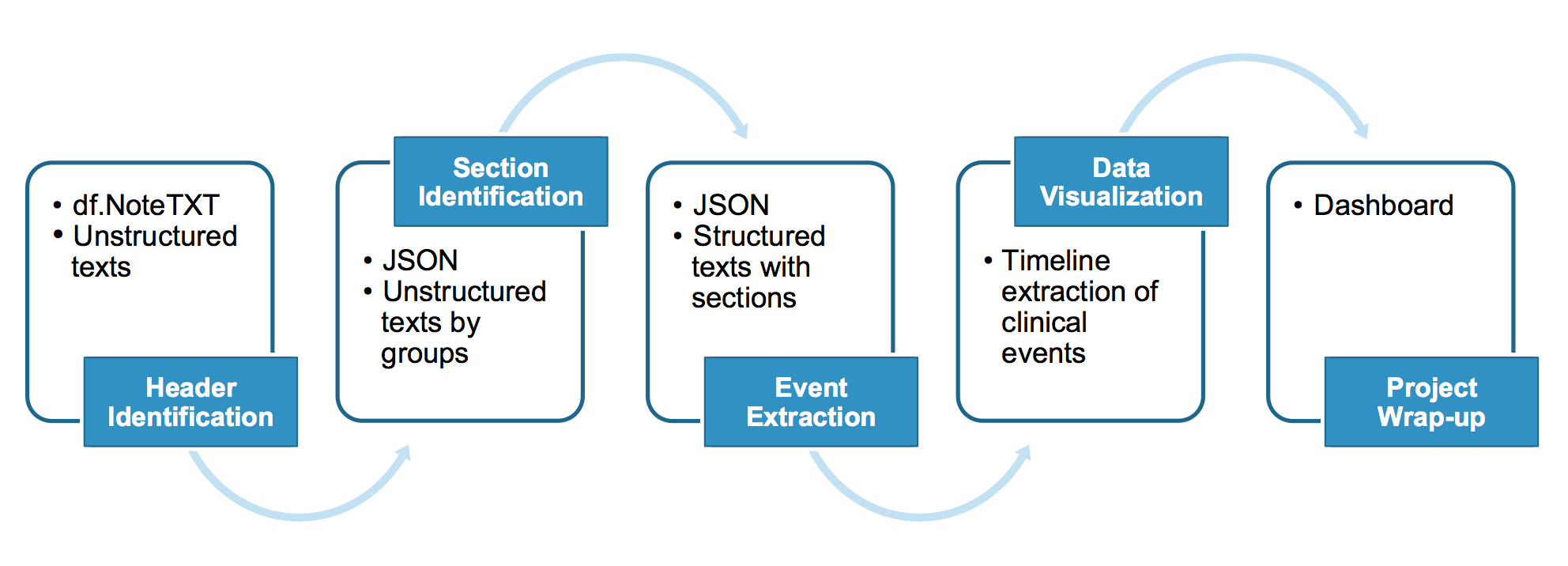
Note Clustering
Utilize the similarity in notes to build up a DBSCAN model
Measuring coherence in notes of each cluster
- Plotting number of sections in a given class
- Average Overlap Count with sections in class regex
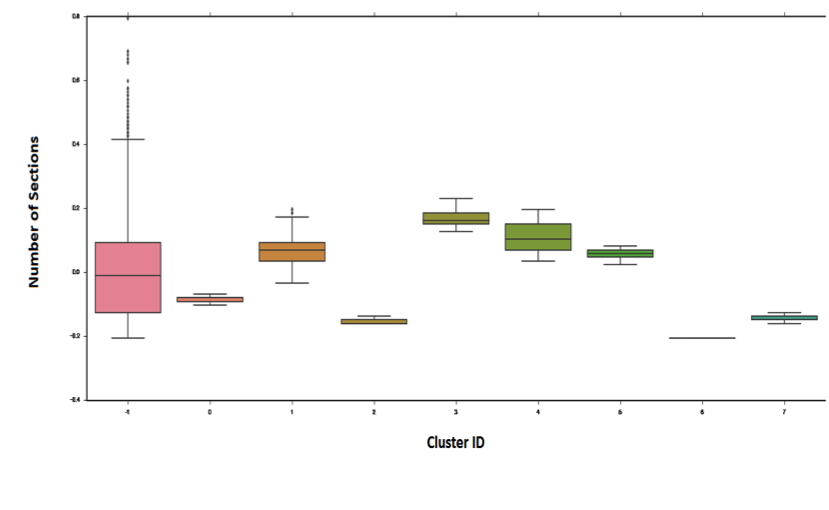
Note Classification
Notes from same institute and department have similar
(1) structure, (2) sections, (3) format
Structure-based Classification
Objective
- Group notes having similar structure
- Classified 52% of notes into 19 classes
Methodology
- Strategically extract tokens from top section of notes
(e.g. SURGEON)
- Have chance to implicitly reveal department
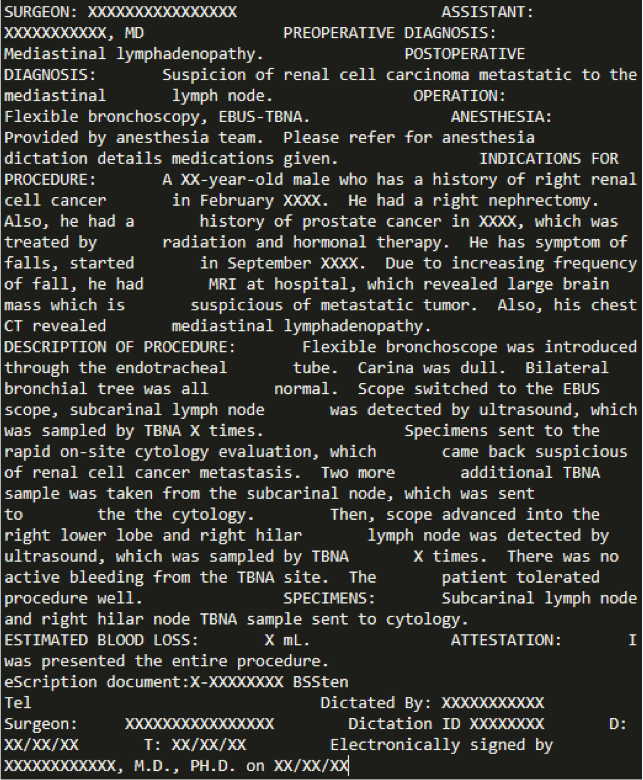
Rule-Based NLP Method
Methodology
- Manually explore notes of a class and find possible sections
- Build sections dictionary, regular expressions
- Parse notes to extract section names
- Append section contents following each section name
Advantages
- Extract predictable sections with full accuracy from well-structured notes
- Successfully processed ~35% notes
Disadvantages
- Requires huge Initial Human Effort
- Fails to capture unpredictable sections
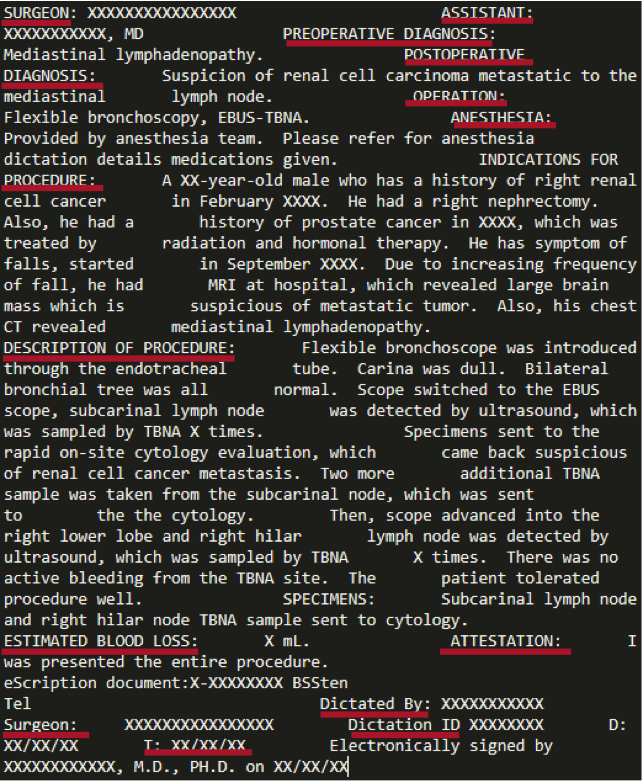
Machine Learning Method
Methodology
(1) Define output values
- Ruled-based identified section headers: True
- Other section candidates: False
(2) Extract section candidates
- N-Grams: Too many candidates
1,2,3-Gram tokens for a note of 1000 tokens, would yield 3000 n-grams
- N-Grams beginning at each sentence: Still too many candidates
1,2,3-Gram tokens for a note of 1000 tokens and 300 sentences would yield 300 n-grams
- POS Tag Chunking: Computationally slow
Extracts chunks of known sections and yields 30% of tokens of above method
(3) Conduct feature selection
- Syntactic analysis
- Semantic analysis
(4) Modeling
- Section Identification -> Binary Classification
- Model selection and evaluation
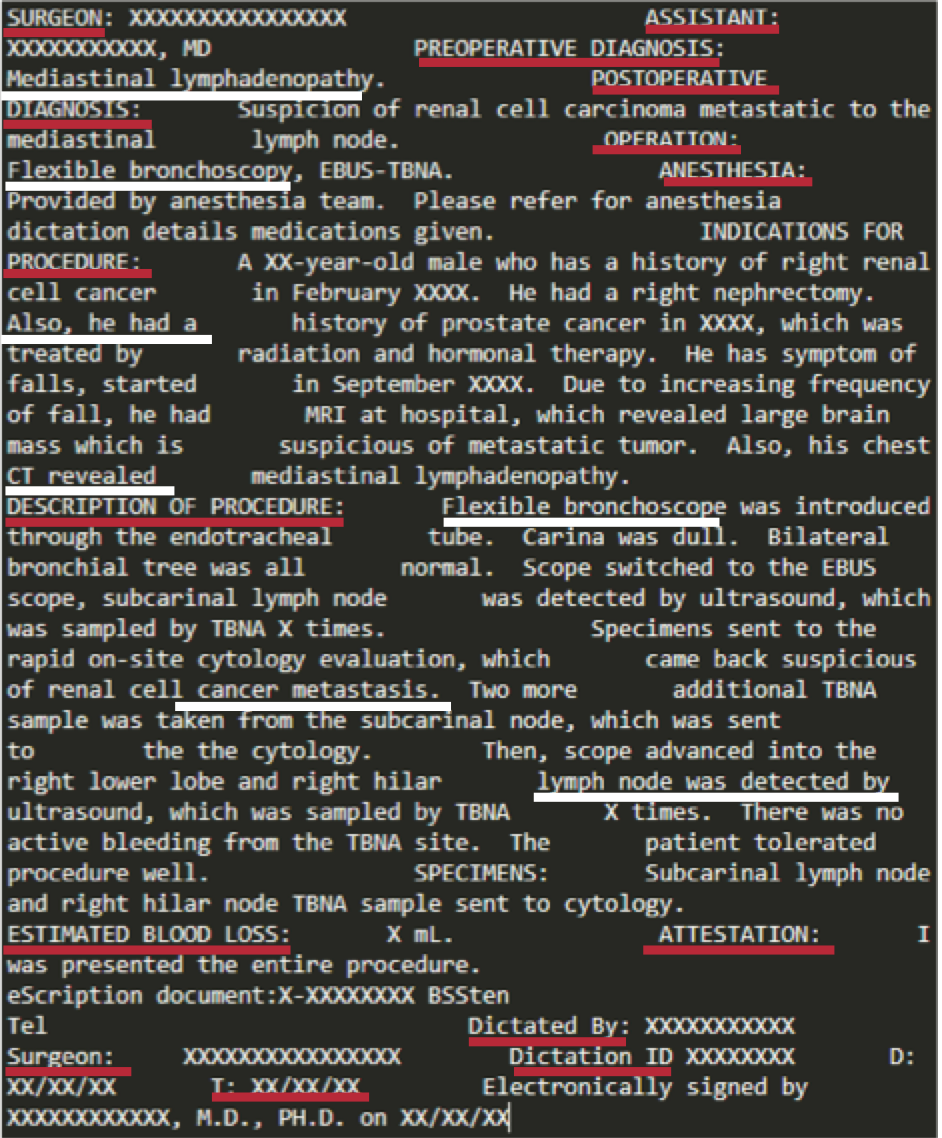
Pos Tag for Sections
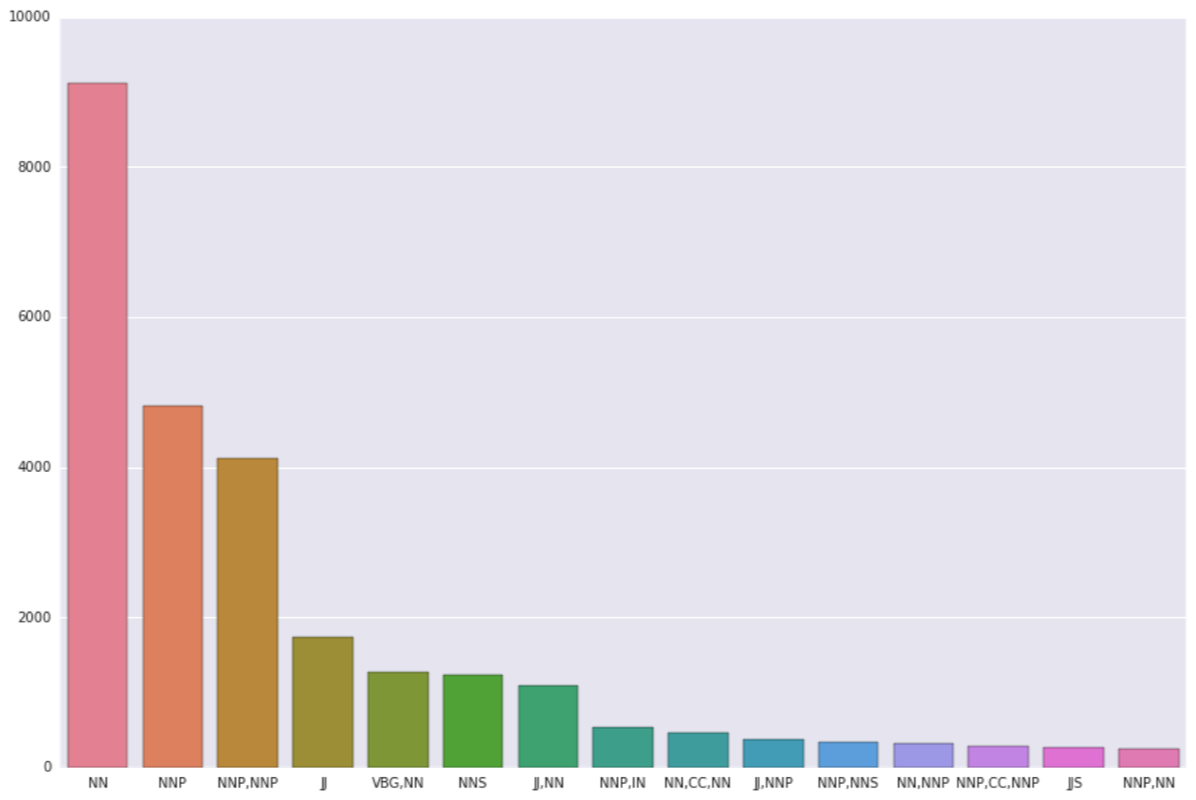
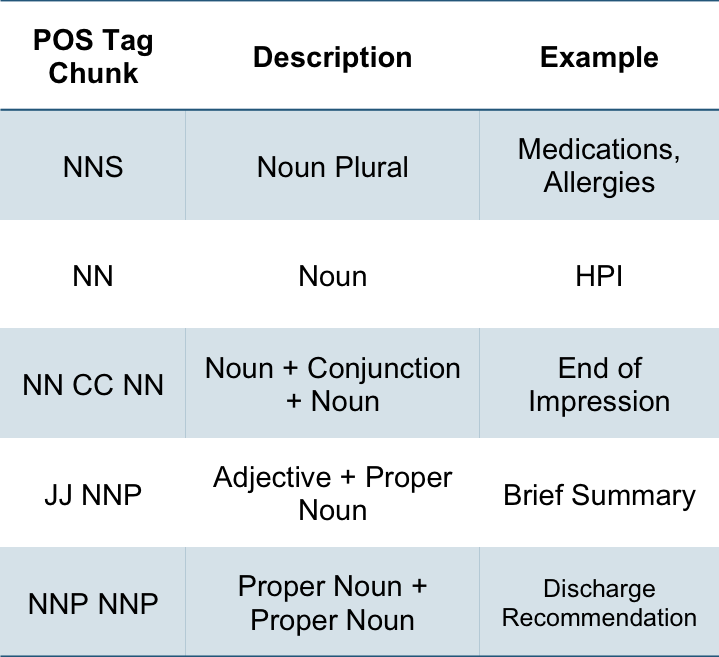
Feature Engineering
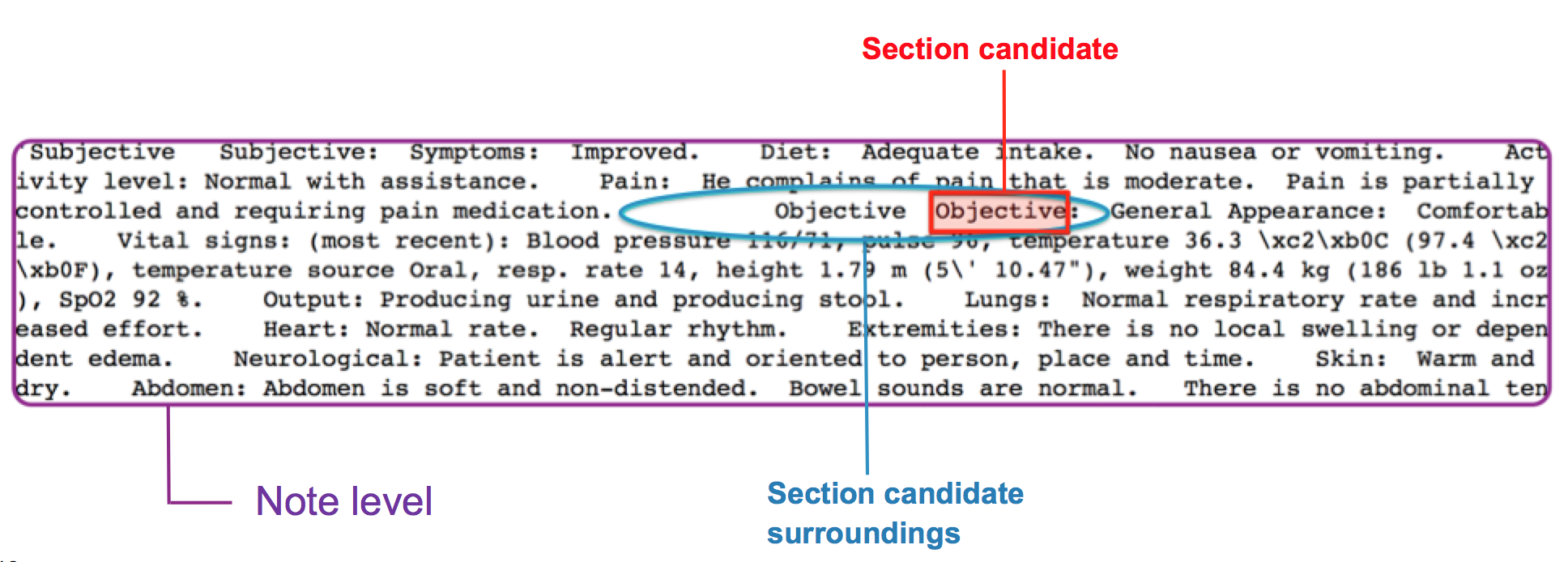
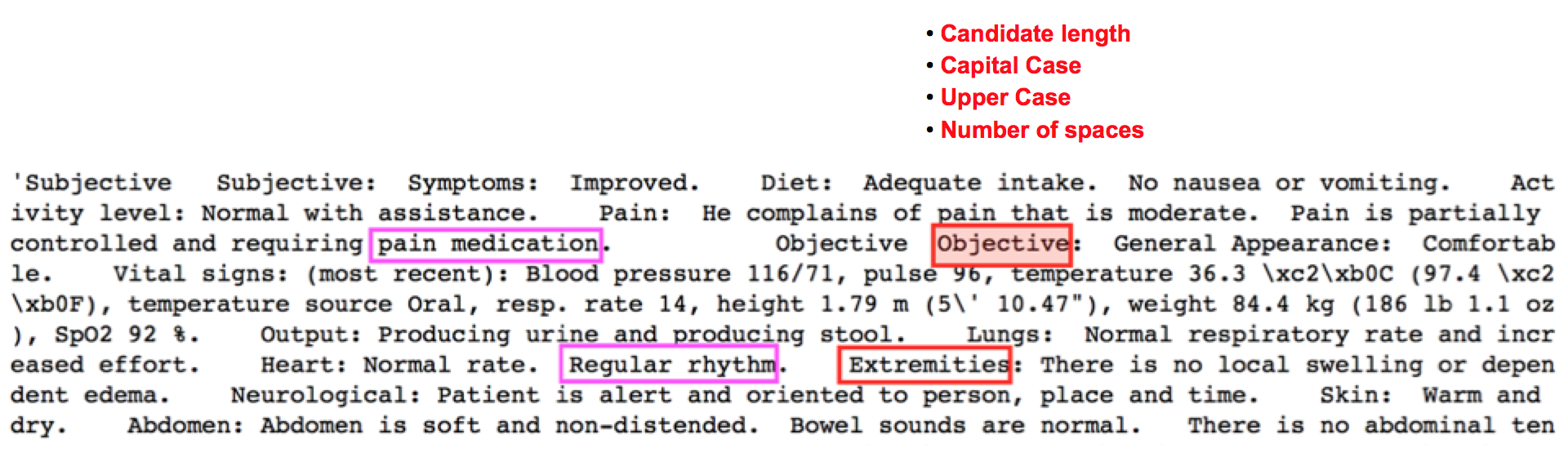
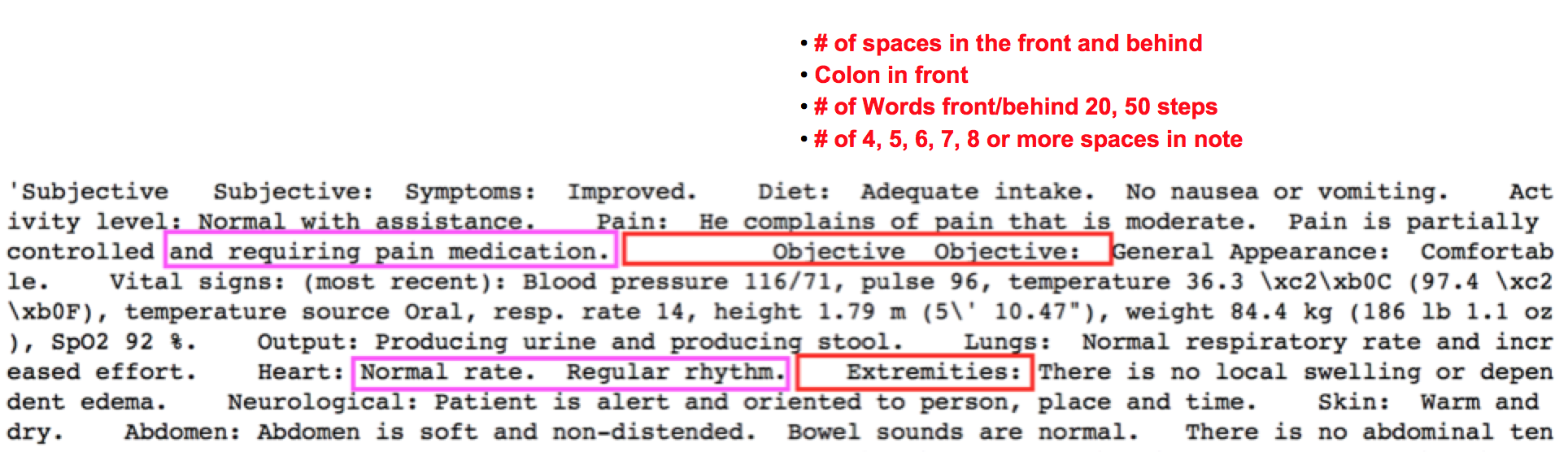
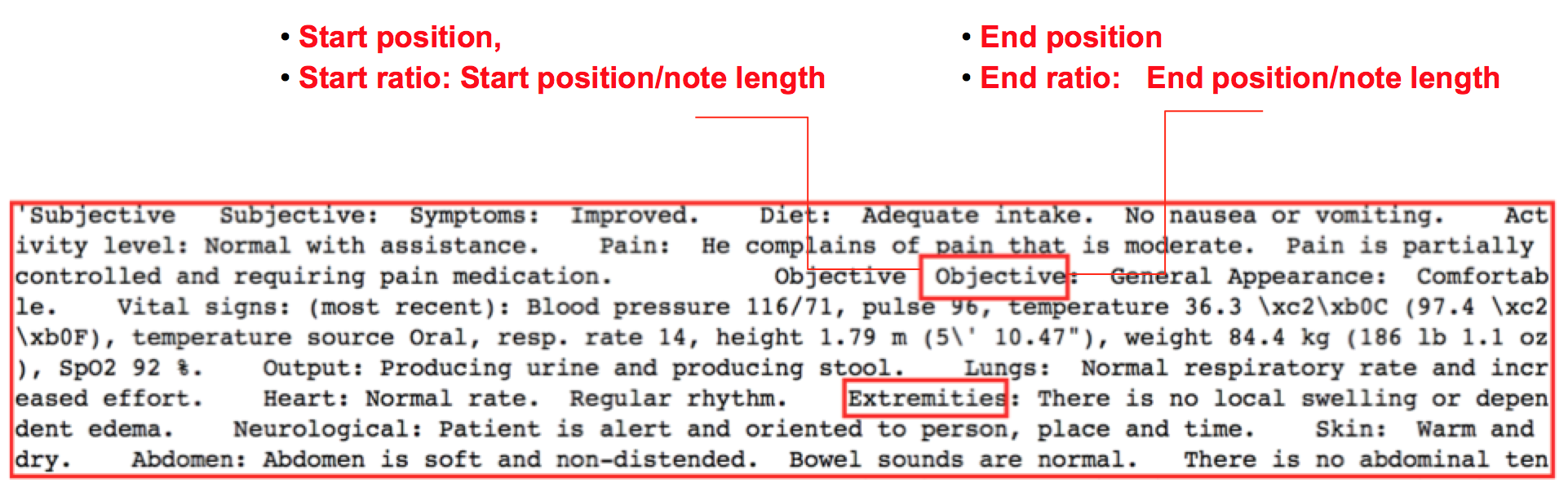
Model Selection and Estimation
Models: Decision Tree, Random Forest, Gradient Boosting and Logistic Regression
Imbalanced Class Problem
- Around 4 million observations
- About 4.3% observations are sections
- Down-sampling non-sections
Results for models using 70-30 train-test split (Training set accounts for 70% of the whole dataset).

Clinical Event Extraction
Convert medical records to a JSON file
- Each patient has multiple medical records
- Each record has record type, header, date, and multiple sections
- Record type: Progress Notes, Discharge Summaries, H&P
- Most frequent section names: INDICATION, SUBJECTIVE, OBJECTIVE, PROCEDURE, Assessment/Plan, Care Management
Extract keywords from section contents
- e.g. extract Staging from Assessment; extract drug names from MEDICATIONS
- Append the events to the JSON file under each note accordingly
Dashboard
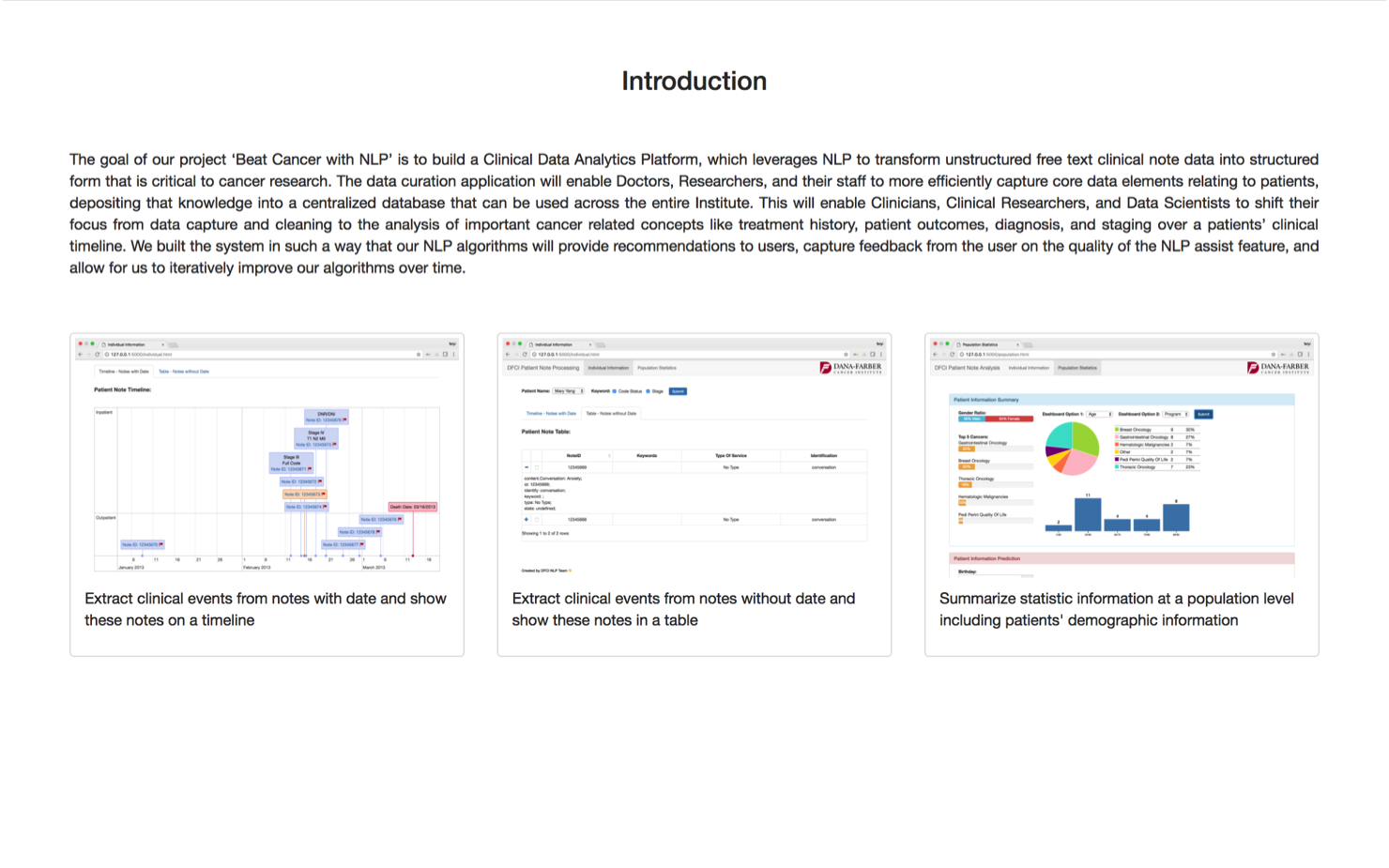
Dashboard
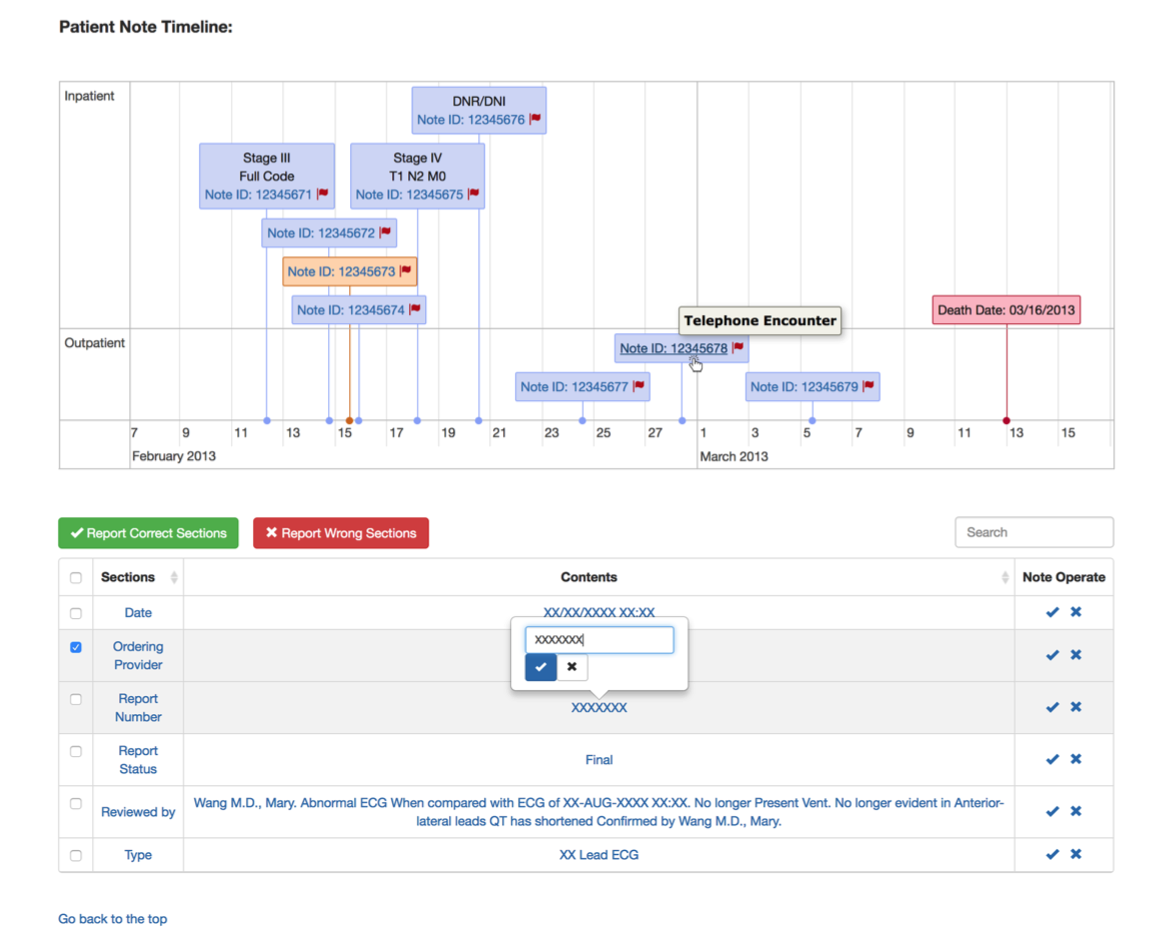
Dashboard
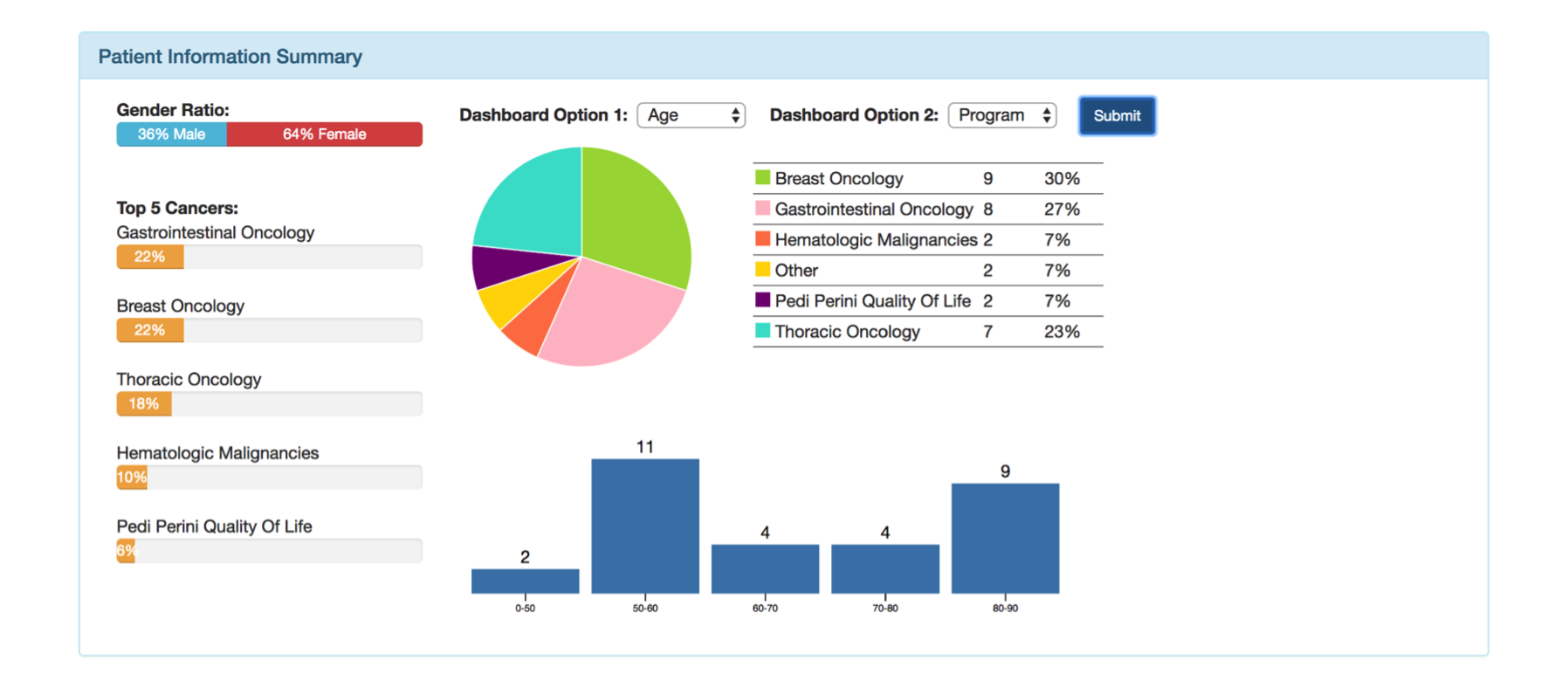
Python Library
- Wrap up analysis modules
- Document the project using Sphinx
- Deploy the dashboard on server
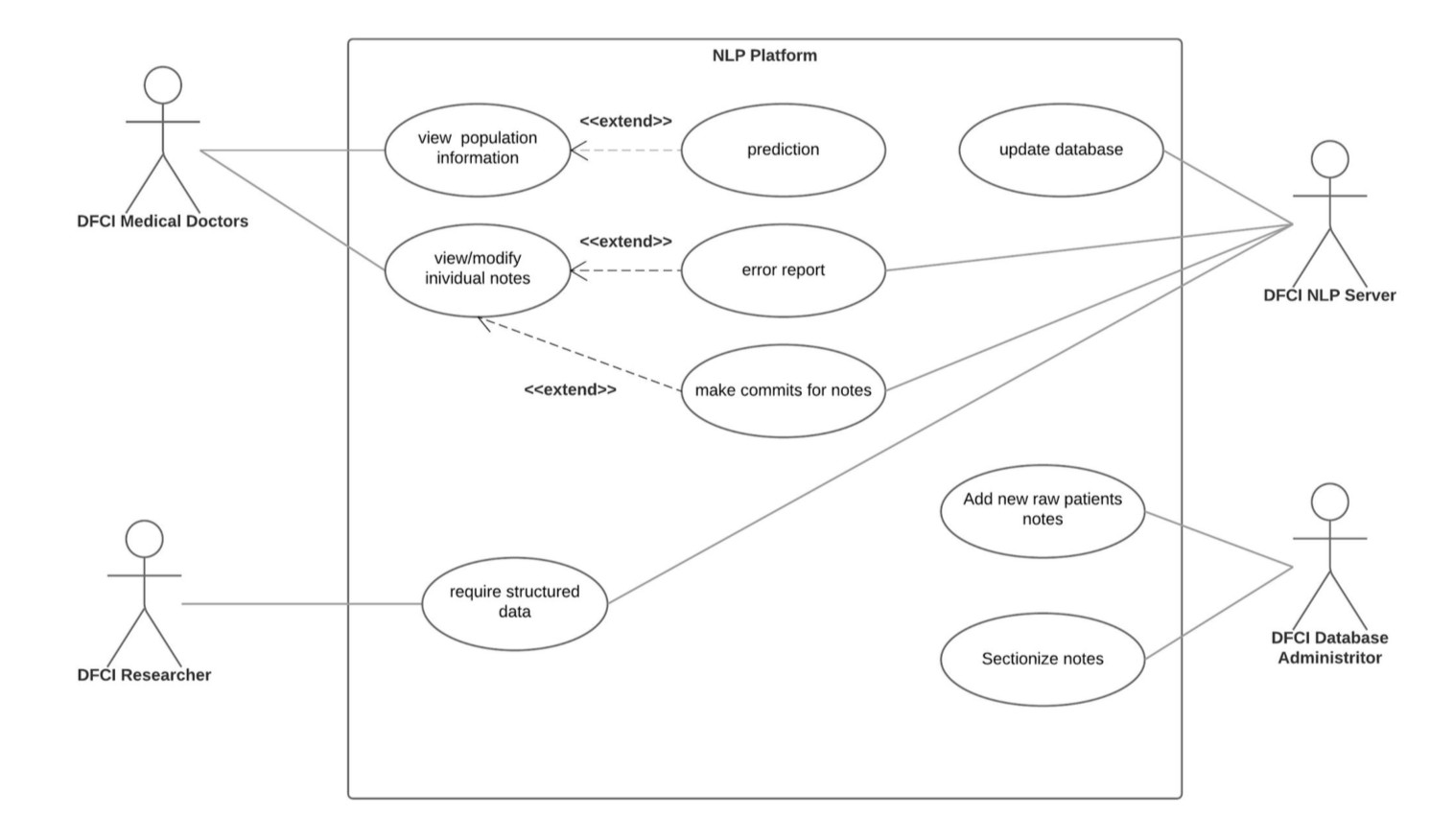
Use Case Diagram